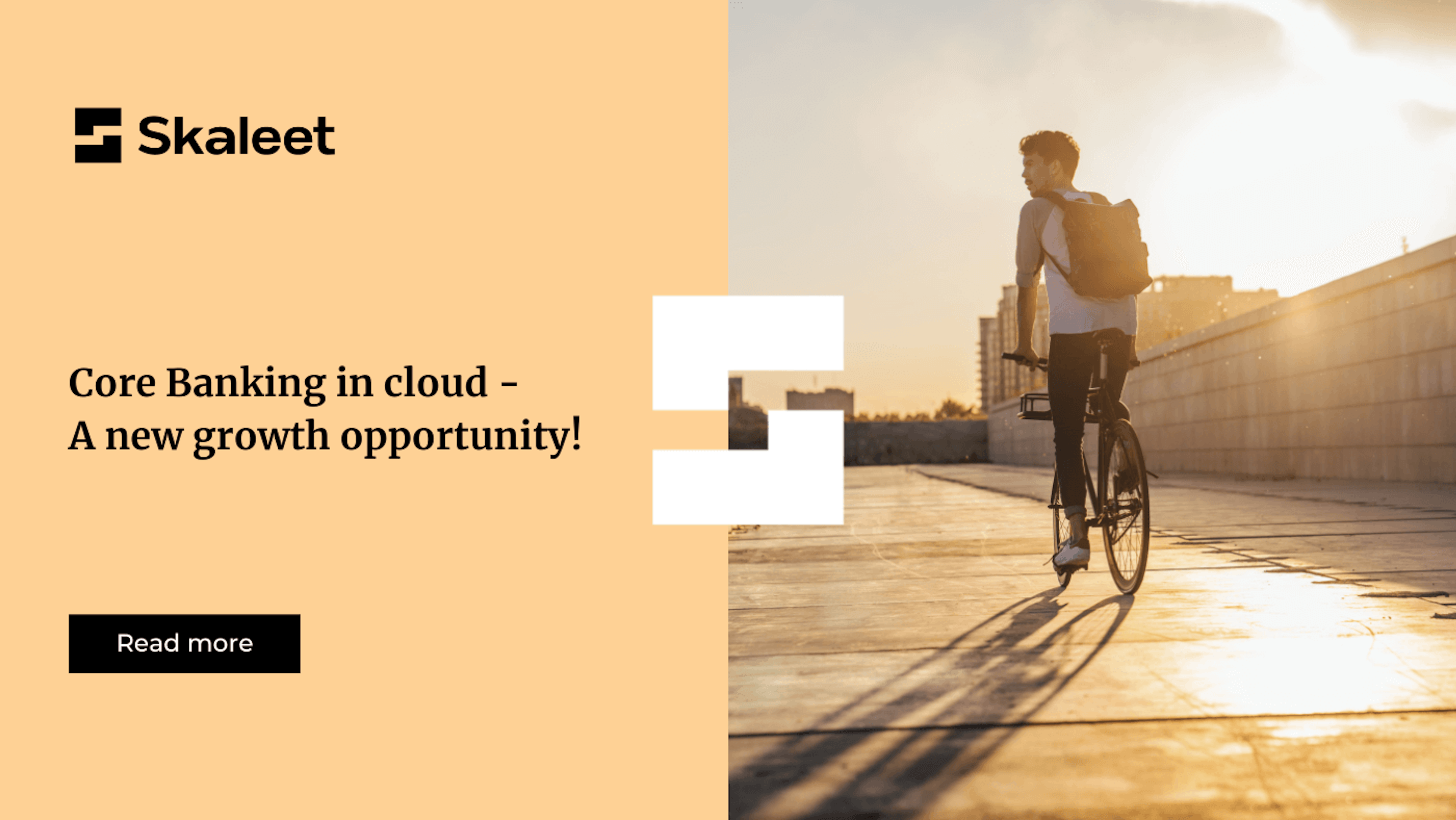
October 18, 2022
Core Banking in cloud - A new growth opportunity! 🚀
Moving to the cloud is a wise move!
Digital disruption was well underway before the Covid pandemic struck, but the pandemic accelerated those trends in ways few could have anticipated in 2019. Now, digitalization has transformed every aspect of life; technologies seen as disruptive pre-pandemic are now the new normal.
Nowhere is this more evident than in the area of finance and consumer lending, thanks to the influence of fintech. Consumers today can access credit in a matter of minutes with just a few taps on their smartphones. And this is increasingly true even for entrepreneurs and small and medium-sized enterprises in the business lending space.
Financial institutions have traditionally been inward-looking and risk-averse, anchored to legacy systems and monolithic infrastructures that limit their ability to respond quickly to changing consumer needs. Their inability to innovate on new solutions and put them where consumers want them in a timely and cost-efficient way opened the door for new entrants to bridge the gap in lending.
Opportunities abound for agile new-to-market lenders that can move from concept to credit offering in a matter of months to meet a market need. The challenge, however, is quickly forming a lending infrastructure and credit-decision platform that enables them to move rapidly while managing credit risk.
New entrants often face technology and infrastructure hurdles in bringing solutions to the market. Challenges such as finding the right data sources, analytics capabilities, and credit-decisioning models can slow the pace of innovation, leaving space for challengers to step in and capitalize on new lending opportunities.
Financial institutions can use the following four-part framework for rapidly developing the next-generation credit-decisioning models that underpin new consumer lending products.
New credit risk models require new sources of data that go beyond credit bureau reports and demographic data. These can include deposit, current account information, and transaction data from other banks, as well as owned, internal data like CRM data, share of wallet and call records, and customer surveys for nonbank lenders.
External data sources such as telecoms, utility companies, insurers, and government agencies can also be leveraged to supplement internal data. For consumers without a documented credit history, mobile phone data can provide a wealth of useful information about bill payments and mobile transactions.
Lending leaders are embracing open banking for a more complete view of their customers since it gives visibility into a customer’s entire banking ecosystem. Because open banking can pull in transaction details across banks, it can serve as the foundation for next-gen credit analytics.
New entrants into the lending market have a distinct advantage over incumbents because they can build an agile, modular engine unencumbered by legacy systems. By using advanced analytics and machine learning, lenders can automate most of the underwriting processes, leading to more accurate decision-making and cost efficiencies.
A modular approach also enables integrated end-to-end risk assessment with the ability to retain control of strategic processes while outsourcing others. This shortens the time to launch a credit engine to as little as six months or less, compared with a year or more for traditional legacy systems.
Lenders can take a buy or build approach to infrastructure depending on their perceived competitive advantage and existing technology. For example, a telecom company launching a cash loan product for existing customers is competing on the basis of its strong customer relationships and therefore may only need a basic turnkey risk assessment solution to bring its solution to market.
On the other hand, a financial institution offering bespoke business financing whose competitive advantage is integrated, tailored lending solutions will need to build a lending infrastructure from the ground up.
Again, a modular approach works best because it allows lenders to build a customized, hybrid infrastructure that suits their risk models and lending solutions.
Lenders only need to create risk models once but they need to carefully monitor them over time to identify warning signs and spot potential problems. This means choosing and tracking key metrics such as the population stability index to measure the customer base against the risk model it was built on, and the credit default rate to measure the health of the portfolio.
Lenders should go beyond simply tracking and monitoring their numbers; they should also compare them with industry benchmarks to identify deviations. Using a dashboard that plugs in these values in real-time helps lenders quickly make corrections to their models and frameworks.
If COVID taught the industry anything, it’s that going forward, risk models must account for the possibility of the financial crisis and other significant disruptions. Developers should always keep in mind that any models they build must be proactive to anticipate data anomalies and major disruptions.
Automated credit decisioning models are at the heart of digital transformation in consumer lending. So how do lenders shrink the two-year timeframe traditional credit decisioning models require? By adopting an agile five-stage process that can have a new credit model up and running in less than six months.
Review and test the methodological setup of the credit model and test its performance to identify potential weaknesses and areas of improvement.
Choose the data sources to include in your scoring model and create a plan for including them. Test the credit model against peers and other segments to suss out any weaknesses.
Format and completeness test the data in preparation for modeling.
Develop and iterate the next-generation credit scoring model for a product-reading MVP. In most cases, this requires three cycles of modeling, analysis, feedback, and adjustment.
To complete the lending transformation, update the credit-decisioning model with the new credit-scoring model and automate lending processes.
Skaleet’s Core Banking Platform uses a microservices architecture to help financial institutions bring new credit solutions to market quickly. The platform uses open APIs to completely digitize the lending journey from application to disbursal.
The Skaleet Core Banking Platform integrates leading market solutions to help new-to-market lenders quickly build a lending infrastructure and credit-decisioning framework. Its data-oriented design enables the collection of structured and unstructured internal and external data needed to build new credit risk models. The addition of advanced AI and scoring solutions lets lenders offer a seamless, personalized customer experience and immediate “time to yes.”
With Skaleet’s partner integrations, lenders can build personalized workflows for innovative consumer credit solutions like BNPL (“Buy Now Pay Later”). Its agile, cloud-native platform helps lenders reduce risks, make faster decisions, and improve the consumer lending experience.
The post–pandemic era opened up exciting opportunities for financial institutions to bridge the gap between traditional consumer lending and the personalized credit solutions today’s digitally native consumers expect. Lenders who can deliver them quickly and at scale will have a clear competitive advantage.
Innovation. FinTech. Digital Banking. Neobanks. Open Banking. Core Banking. Cloud.
October 18, 2022
Moving to the cloud is a wise move!
Register to our newsletter